In a previous post, we discussed the importance of data modelling and the implementation of a robust enterprise data model. An aspect also often forgotten is data governance.
What we saw in the past was that companies were urging for a rapid implementation of a data platform and making sure as much data was loaded into this platform in the least amount of time, often neglecting adequate attention to data governance.
In short, data governance encompasses the instructions, the procedures and responsibilities you put in place to ensure your data is and remains secure, accurate, high-quality and easily maintainable. A lack of proper data governance strategy increases the risk of security vulnerabilities, poor data quality, and maintenance difficulties in your data platform.
Within togaether, we guide our customers through the implementation of key data governance aspects, including:
- Data quality
Investing in a modern data platform is essential for enabling the entire organization to derive insights and make informed decisions while looking all through the same window. However, if the data quality of the data within the data platform is low, the insights derived from this data may be inaccurate, resulting in a lack of trust in the platform.
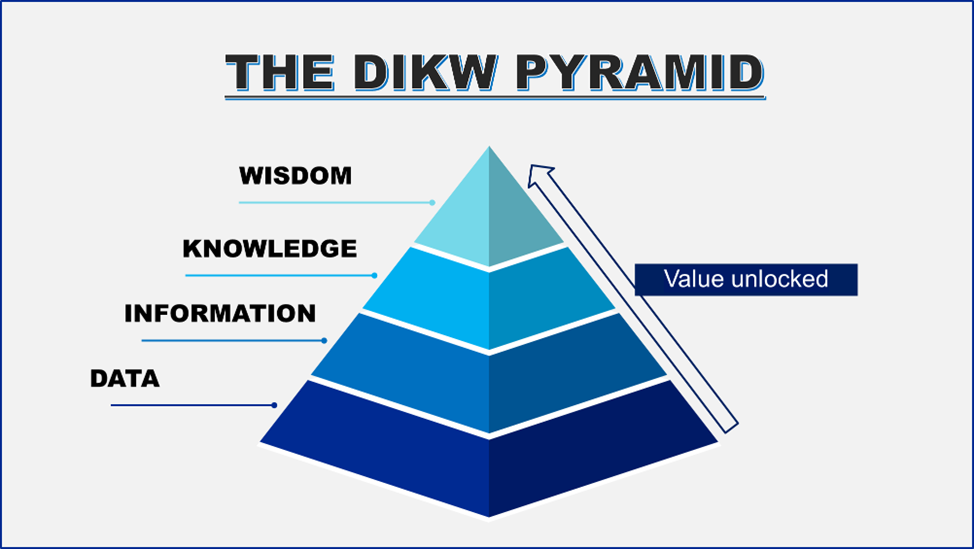
- Data in its raw formation is a combination of 1 and 0’s, meaningless without context.
- When context is added to data, it will become information. Information can be used to answer simple questions like when, who, what, where.
- Add meaning to information and it becomes knowledge, knowledge creates understanding for questions like how and why.
- When knowledge is used to create insights, well informed decisions can be made resulting in wisdom.
It’s fundamental to acknowledge that the integrity of this pyramid hinges on the quality of its foundation—the data layer. When data quality is low, information becomes unreliable, knowledge gathering is incorrect or insufficient and the trust in creating wisdom is low. This will result in limited collaboration through data.
This functional approach applies also to modern data platforms: when data quality in these platforms is low, all the effort put into the creation of such a platform becomes useless. Therefore, apart from investing in a state-of-the-art data platform, it is also essential to invest in policies and frameworks aimed at enhancing the data quality within your organization.
The following topics give some more insights into enhancing the data quality within your organization’s data platform:
Data profiling: this process aims to extract the best quality information and insights from a specific set of data, enabling organizations to make informed decisions to reach the organization’s objectives. As the volume of data continues to grow, maintaining good data quality becomes challenging for companies, impacting their ability to work efficiently with data.
Data quality metrics: These metrics critically evaluate data’s correctness, completeness, consistency, timeliness, and reliability. Establishing standards and conducting regular assessments helps track progress and identify areas for improvement.
Data Validation and cleansing pipelines: implement validation checks to ensure that data adheres to defined rules and standards. Apply cleansing processes to correct errors, inconsistencies, or inaccuracies in the data.
Apart from a separate data quality tool, it is also perfectly possible to embed data validation and cleansing rules in the data pipelines of the Data Lakehouse. Once data is ingested into the RAW layer, data will be transformed and modeled in order to be fully optimized for analytical purposes.
- Data Ownership
Data ownership signifies the control over and accountability for data or information. This concept is is important in data governance because it helps to ensure that the data is and remains accurate, reliable, and secure, which ensures that value can be derived from its use.
When there is no data ownership, it becomes challenging to implement proper data governance as there is no accountability nor responsibility for the data.
- Data security
Securing data in a modern data platform is a crucial element that requires the implementation of measures to ensure the confidentiality, integrity, and accessibility of data.
A cloud that isn’t secure will fail to exist in the future. It’s imperative that internal security measures of cloud services are maintained at the highest standard.
Noteworthy is that the security you implement will be your own responsibility. Which means you must implement security policies that best fit your company.
When securing a data platform, following aspects need to be considered:
Encryption: When storing data (in rest) in a database or data lake, data will be encrypted. All Cloud vendors have the capabilities to use Zero-knowledge encryption.
Access control policies: When setting up access control policies, it is important to create roles and responsibilities to define who can access, modify or delete data within your data platform. Implement robust access controls to ensure that only authorized users have access to specific data and functionalities within the data platform.
Access control systems: In a data access control policy, the access control systems are the methodologies that enforce the access privileges defined in the policy. These systems dictate how access is granted, regulated, and restricted. They form the backbone of the policy, defining how to implement access controls across an organization.
User access Management: To effectively manage data access, user access needs management throughout the entire employment lifecycle. User access management defines the processes for granting new access rights, auditing user access, and revoking user access.
Data masking/anonymization: Apply data masking techniques to conceal sensitive information from users who do not need to see the complete dataset. Use data anonymization methods to replace personally identifiable information (PII) with fake or masked data to protect individual privacy.
- Data Management
Data management is a set of tools and processes designed to ensure your organization maintains complete control over its data assets. Below are the key aspects of data management that we consider essential for effective data governance.
Data Glossary: the primary purpose of a data glossary (often referred to as a business glossary) is to establish a common language and understanding of data across different teams and departments. It promotes data governance, data quality, and data consistency by ensuring that everyone involved with the data uses the same definitions and interpretations for specific terms.
Data Catalog: a data catalog is a centralized and organized inventory or repository of metadata and information about the data assets within an organization. It serves as a comprehensive reference guide that provides users with insights into available data sources, their structure, contents, and other relevant information. The main purpose of a data catalog is to facilitate data discovery, data governance, and data management processes.
Data lineage: data lineage is the record or documentation that traces the origin, transformation, and movement of data from its source to its destination or final output. It provides a detailed understanding of how data flows through various systems, processes, and transformations within an organization. Data lineage is an essential aspect of data governance and data management, as it ensures data quality, data accuracy, and regulatory compliance.
Master Data Management: Master Data Management (MDM) is a comprehensive process and a set of technologies employed to ensure the consistency, accuracy, and centralized maintenance of an organization’s critical data, referred to as “master data“.
The primary objective of MDM is to establish a unified, authoritative, and dependable version of master data throughout the entire organization, regardless of the various systems or applications that store the data.
The MDM process involves identifying and defining the essential data entities, implementing data governance rules and policies, and deploying tools and technologies to effectively manage and synchronize the master data across diverse systems.
- Data modeling
As outlined in a previous post, data modeling is a critical component of Data Governance, involving the implementation of an enterprise data model.
We will not go into detail anymore, but we want to focus on 1 part: a common oversight in data modeling is the absence of a comprehensive enterprise data model. This lack of proper documentation or accountability can lead to fragmented data environments, where models operate in silos, complicating maintenance and coherence.
However, it’s important to say that, in some cases, it can be a strategy for not going for an enterprise data model but having several data models next to each other – it all depends on the needs of the customer.
At togaether, we believe these Data Governance principles are fundamental to the successful implementation of a modern data platform. Using our expertise and guidance, we are able to assist our customers in making the right decisions for the implementation of the above aspects and work out every single detail that resides in each of the above-described aspects.
Want to know more? Reach out to us and we are more than happy to assist you with the implementation of a proper Data Governance strategy!